SOLUTIONS
Drug discovery services
Address the critical challenges associated with the drug discovery pipeline using our core technologies based on simulations and machine learning.
Our private state-of-the-art GPU cluster
Security certified by ISO 27001 (expected during 2023)
100% carbon-neutral electricity sources
Fast progress using our technology platform and our team of medicinal and computational chemists
More than 16 years of experience using computational chemistry for drug discovery
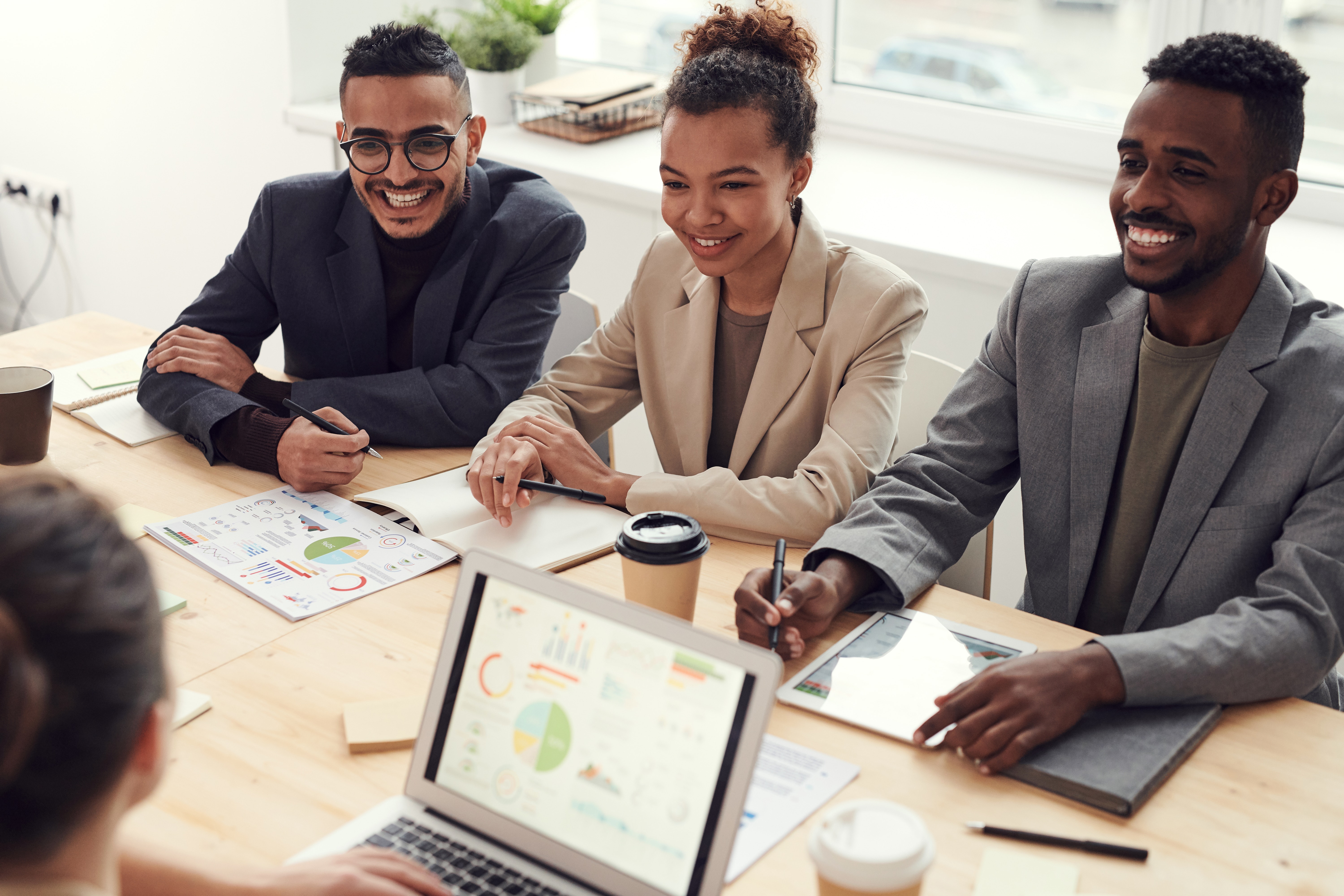
Cryptic Pockets and Fragments Scouting
This protocol simulates the target of interest (GPCR, kinase, ion channel, etc.) in a solution of water and a proprietary library of chemical probes to characterize the protein surface druggability.
Revealing binding hotspots. These hotspots have been proven to correlate very well with actual pockets.
Binding modes. The probes prove very valuable to inform the binding modes of drug-like molecules
Druggability. Explore which pockets are druggable and the most relevant residues.
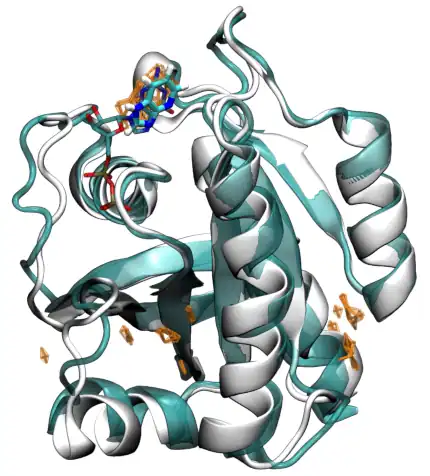

Virtual Screening
Screening allows for reducing the compounds which need to go to experimental testing. This protocol won 2 blind sub-challenges of the D3R Grand Challenge 4.
Free docking: No restrictions or prior knowledge is imposed in the docking exercise. Poses are re-scored with KDEEP.
Scaffold-hopping: Identify compounds in your library with great pharmacophoric overlap against a known binder.
Template docking: Use a known binder to guide the docking of a similar compound or series.
Constrained docking: Impose restrictions so certain moieties in the ligand (e.g. aromatic rings) are placed in the right spot.
In Silico Conformational Assay (ISCA)
This protocol allows to be beyond crystal structures revealing the conformational landscape of a target and structural insights which can directly impact a drug discovery campaign.
Unique structural insights: Knowing the different conformations that your target can adopt can give you an edge.
4D structures: Build an ensemble of pocket conformations.
Understanding transitions: Visualize how a virus spike protein adopts the host-bound-ready state or how a GPCR goes from inactive to active.
Estimate conformational stability: Differentiate between the wild-type and a mutant structurally.
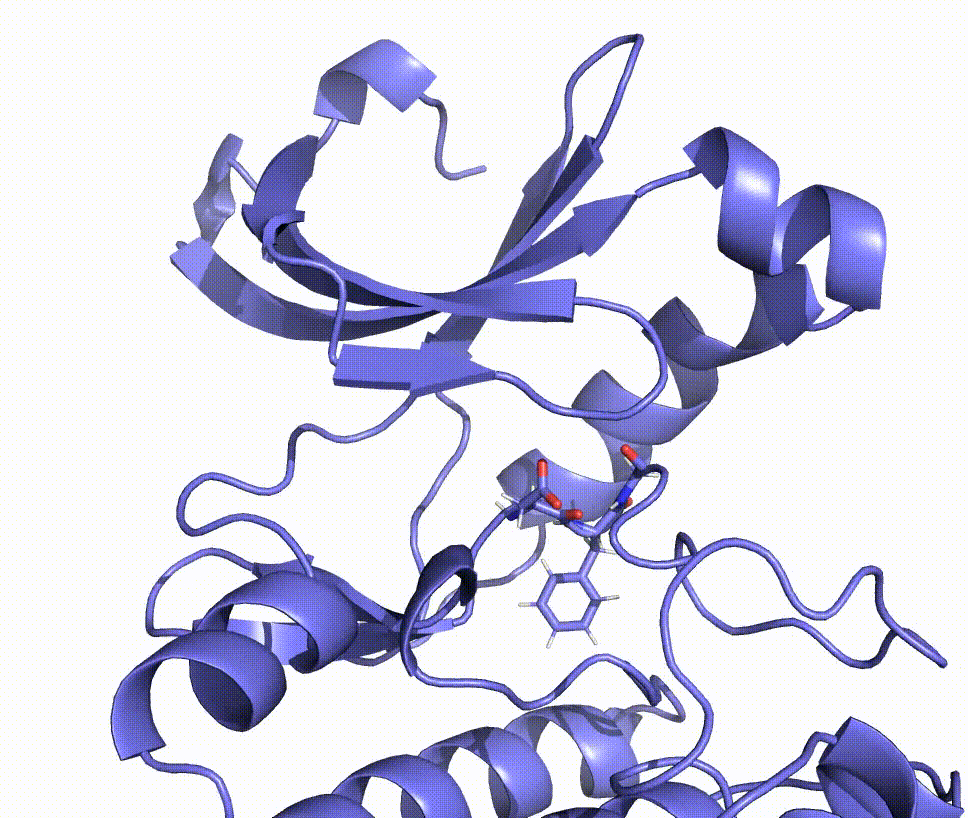
In Silico Binding Assay (ISBA)
This protocol replicates in-silico the process of molecular recognition between a drug and its target revealing structural insights as well as its kinetics and thermodynamcs.
In a collaboration with Pfizer, ISBA succeded in resolving the binding process where docking failed to produce a pose that could explain the behavior of some mutants.
In a collaboration with Pfizer, ISBA succeded in resolving the binding process where docking failed to produce a pose that could explain the behavior of some mutants.
Rational drug design: Knowing the binding mode of a ligand is the first step towards structure-based design and lead-optimization.
Estimate binding affinity and kinetics: Predict the binding kinetics (Koff and Kon) of a ligand with Markov state models.
Binding pathway: Binding modes and pathways with molecular dynamics Obtain the pathway that the ligand follows from bulk to bound state.
Deep Learning Molecular Properties
We pioneered the use of deep learning in structural biology. Using our TorchMD-net framework, we leverage state-of-the-art equivariant machine learning to predict properties like binding affinities.
Validated on data from pharmaceutical companies and in blind challenges. KDeep won two blind subchallenges of the D3R Grand Challenge 4.
Validated on data from pharmaceutical companies and in blind challenges. KDeep won two blind subchallenges of the D3R Grand Challenge 4.
DeltaDelta: Relative binding affinity predictor for regression of congeneric series
KDeep: Binding affinity classification and regression for screening. Able to screen multi-million datasets in hours.
KDeep2D: The ligand-based predictor reached the second highest rank in the Sampl challenge.
KDeep-Trainer: Retraining on internal datasets drastically improves the reliability of prediction reaching high correlation similar to MD-based methods with data on approximately 50-100 molecules.
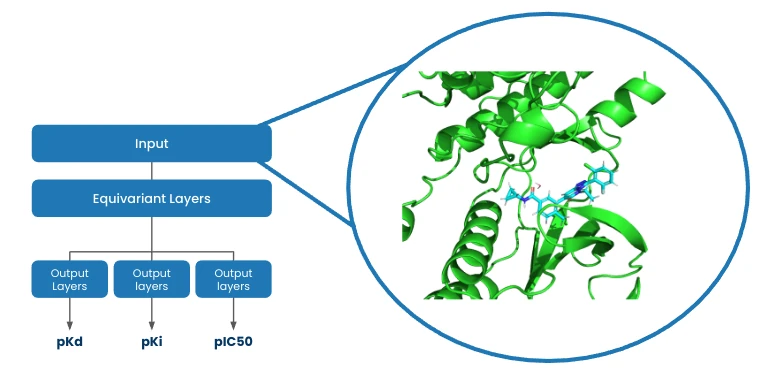

De-Novo and Decorative Library Generation
Acellera’s chemical language model guided by a state-of-the-art reinforcement learning algorithm. With AceGen virtual libraries of chemical compounds can be rapidly generated while optimizing multiple objectives.
Chemical space exploration: Navigate effectively through chemical space using optimization metrics
Targeted library generation: Produce target-optimized libraries using QSAR models. De-novo and decorative design modes.
Scaffold decoration: Optimize your hit while preserving its core.
Relative Binding Affinity Prediction
Relative binding free energy (RBFE) is widely used in drug discovery to identify potential drug candidates and optimize lead compounds.
Lead Optimization: Optimize lead compounds by predicting how changes to a ligand’s chemical structure affect its binding affinity.
Quantum accuracy: Our method uniquely offers quantum level accuracy by the use of machine learning potentials trained on quantum calculations.
Understanding the SAR: Gain insights into the molecular mechanisms of ligand binding and help researchers design new compounds with improved binding affinity.
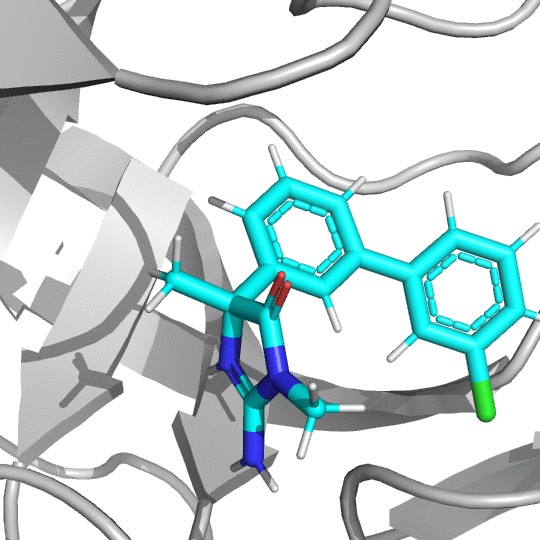
GET IN TOUCH