research & Development
Advancing Our Mission
Our research and development efforts are aimed at advancing our mission to transform drug discovery into a high-accuracy computable task.
We work closely with both public and private institutions, and we frequently publish our findings in reputable academic journals. In addition, we have initiated and continue to support various open access science and open source software projects.
We are always open to considering new collaborations.
We work closely with both public and private institutions, and we frequently publish our findings in reputable academic journals. In addition, we have initiated and continue to support various open access science and open source software projects.
We are always open to considering new collaborations.
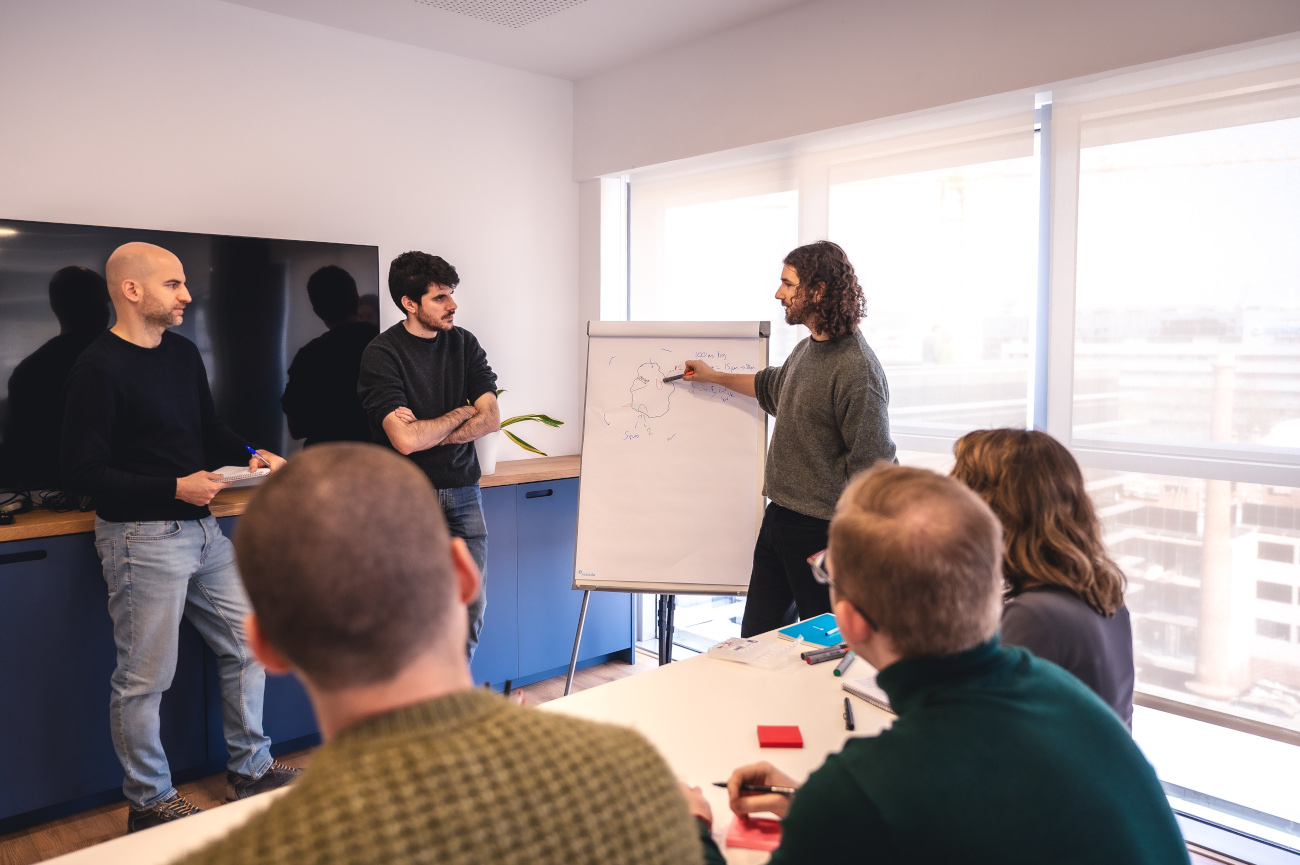

Open source projects
Molecular Modelling For Everyone
We lead and contribute to a number of open source molecular modeling and manipulation projects such as HTMD, MoleculeKit, OpenMM, ACEGEN, TorchMD and TorchMD-Net.
Open access
PlayMolecule.org – An Open Molecular Discovery Toolkit
PlayMolecule features a number of hosted apps for molecular discovery including a powerful online molecular viewer, molecular preparation tools, and binding affinty affinity prediction tools.
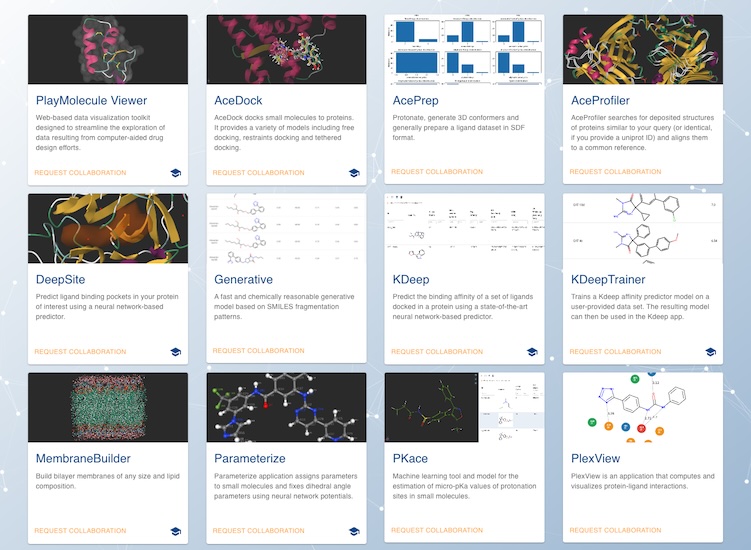
Publications
>11,000
Papers citing Acellera's founders
>900
Papers citing ACEMD software
18
Years of know-how in computational drug discovery
Today, our software ACEMD has over 939 citations, and KDeep and Deepsite are among the most cited papers in the field of ML applied to drug discovery.
Showing
0
results of
0
publications.
PlayMolecule pKAce: Small Molecule Protonation through Equivariant Neural Networks
arXiv preprint
,
2024
PlayMolecule Viewer: a toolkit for the visualization of molecules and other data
Journal of Chemical Information and Modeling
,
2024
Applications
https://doi.org/10.1021/acs.jcim.3c01776
TorchMD-Net 2.0: Fast Neural Network Potentials for Molecular Simulations
Journal of Chemical Theory and Computation
,
2024
Applications
https://doi.org/10.1021/acs.jctc.4c00253
ACEGEN: Reinforcement learning of generative chemical agents for drug discovery
arXiv preprint arXiv:2405.04657
,
2024
Applications
https://doi.org/10.48550/arXiv.2405.0465
Enhancing Protein–Ligand Binding Affinity Predictions Using Neural Network Potentials
Journal of Chemical Information and Modeling
,
2024
Applications
https://doi.org/10.1021/acs.jcim.3c02031
Openmm 8: Molecular dynamics simulation with machine learning potentials
The Journal of Physical Chemistry B
,
2023
Molecular Simulations
https://doi.org/10.1021/acs.jpcb.3c06662
Validation of the Alchemical Transfer Method for the Estimation of Relative Binding Affinities of Molecular Series
Journal of chemical information and modeling
,
2023
Applications
https://doi.org/10.1021/acs.jcim.3c00178
NNP/MM: Accelerating molecular dynamics simulations with machine learning potentials and molecular mechanics
Journal of chemical information and modeling
,
2023
ACEMD/HTMD/AceCloud
https://doi.org/10.1021/acs.jcim.3c00773
Top-Down Machine Learning of Coarse-Grained Protein Force Fields
Journal of Chemical Theory and Computation
,
2023
Molecular Simulations
https://doi.org/10.1021%2Facs.jctc.3c00638
Validation of the Alchemical Transfer Method for the Estimation of Relative Binding Affinities of Molecular Series
Journal of Chemical Information and Modeling
,
2023
Applications
https://doi.org/10.1021/acs.jcim.3c00178
Machine learning coarse-grained potentials of protein thermodynamics
Nature Communications
,
2023
Molecular Simulations
https://doi.org/10.1038/s41467-023-41343-1
Machine Learning Small Molecule Properties in Drug Discovery
Artificial Intelligence Chemistry
,
2023
Molecular Simulations
https://doi.org/10.1016/j.aichem.2023.100020
TorchRL: A data-driven decision-making library for PyTorch
ICLR 2024, arXiv preprint arXiv:2306.00577
,
2023
Applications
https://doi.org/10.48550/arXiv.2306.00577
Structure based virtual screening: Fast and slow
Wiley Interdisciplinary Reviews: Computational Molecular Science
,
2022
Protein-Ligand Binding
https://doi.org/10.1002/wcms.1544
PlayMolecule glimpse: Understanding protein–ligand property predictions with interpretable neural networks
Journal of chemical information and modeling
,
2022
Applications
https://doi.org/10.1021/acs.jcim.1c00691
TorchMD-NET: Equivariant Transformers for Neural Network based Molecular Potentials
International Conference on Learning Representations
,
2022
Applications
https://doi.org/10.48550/arXiv.2202.02541
TorchMD: A deep learning framework for molecular simulations
Journal of chemical theory and computation
,
2021
Applications
https://doi.org/10.1021/acs.jctc.0c01343
AdaptiveBandit: A Multi-armed Bandit Framework for Adaptive Sampling in Molecular Simulations
J. Chem. Theory Comput.
,
2020
Applications
https://doi.org/10.1021/acs.jctc.0c00205
Small molecule modulation of intrinsically disordered proteins using molecular dynamics simulations
Journal of Chemical Information and Modeling
,
2020
Applications
https://doi.org/10.1021/acs.jcim.0c00381
Characterization of partially ordered states in the intrinsically disordered N-terminal domain of p53 using millisecond molecular dynamics simulations
Scientific reports
,
2020
Applications
https://doi.org/10.1038/s41598-020-69322-2
SkeleDock: a web application for scaffold docking in PlayMolecule
Journal of Chemical Information and Modeling
,
2020
Applications
https://doi.org/10.1021/acs.jcim.0c00143
PlayMolecule CrypticScout: predicting protein cryptic sites using mixed-solvent molecular simulations
Journal of Chemical Information and Modeling
,
2020
Applications
https://doi.org/10.1021/acs.jcim.9b01209
Shape-based generative modeling for de novo drug design
Journal of chemical information and modeling
,
2019
Applications
https://doi.org/10.1021/acs.jcim.8b00706
Reconstruction of apo A2A receptor activation pathways reveal ligand-competent intermediates and state-dependent cholesterol hotspots
Scientific Reports
,
2019
Applications
https://doi.org/10.1038/s41598-019-50752-6
DeltaDelta neural networks for lead optimization of small molecule potency
Chemical science
,
2019
Applications
https://doi.org/10.1039/C9SC04606B
LigVoxel: inpainting binding pockets using 3D-convolutional neural networks
Bioinformatics
,
2019
Applications
https://doi.org/10.1093/bioinformatics/bty583
From target to drug: generative modeling for the multimodal structure-based ligand design
Molecular pharmaceutics
,
2019
Applications
https://doi.org/10.1021/acs.molpharmaceut.9b00634
A Scalable Molecular Force Field Parameterization Method Based on Density Functional Theory and Quantum-Level Machine Learning
Journal of chemical information and modeling
,
2019
Applications
https://doi.org/10.1021/acs.jcim.9b00439
PathwayMap: molecular pathway association with self-normalizing neural networks
Journal of chemical information and modeling
,
2018
Applications
https://doi.org/10.1021/acs.jcim.8b00711
PlayMolecule BindScope: Large scale CNN-based virtual screening on the web
Bioinformatics
,
2018
Applications
https://doi.org/10.1093/bioinformatics/bty758
Dopamine D3 receptor antagonist reveals a cryptic pocket in aminergic GPCRs
Scientific reports
,
2018
Protein-Ligand Binding
https://doi.org/10.1038/s41598-018-19345-7
Molecular-simulation-driven fragment screening for the discovery of new CXCL12 inhibitors
Journal of chemical information and modeling
,
2018
Fragment Based Drug Discovery
https://doi.org/10.1021/acs.jcim.7b00625
K deep: protein–ligand absolute binding affinity prediction via 3d-convolutional neural networks
Journal of chemical information and modeling
,
2018
Applications
https://doi.org/10.1021/acs.jcim.7b00650
Drug discovery and molecular dynamics: methods, applications and perspective beyond the second timescale
Current topics in medicinal chemistry
,
2017
Applications
https://doi.org/10.2174/1568026617666170418121951
PlayMolecule ProteinPrepare: a web application for protein preparation for molecular dynamics simulations
Journal of chemical information and modeling
,
2017
Applications
https://doi.org/10.1021/acs.jcim.7b00190
Optimizing Proteins and Ligands for Computerized Drug Discovery
,
2017
Applications
https://doi.org/10.3390/mol2net-03-05072
Dimensionality reduction methods for molecular simulations
arXiv preprint arXiv:1710.10629
,
2017
Molecular Simulations
https://doi.org/10.48550/arXiv.1710.10629
DeepSite: protein-binding site predictor using 3D-convolutional neural networks
Bioinformatics
,
2017
Applications
https://doi.org/10.1093/bioinformatics/btx350
High-throughput automated preparation and simulation of membrane proteins with HTMD
Journal of Chemical Theory and Computation
,
2017
ACEMD/HTMD/AceCloud
https://doi.org/10.1021/acs.jctc.7b00480
Complete protein–protein association kinetics in atomic detail revealed by molecular dynamics simulations and Markov modelling
Nature chemistry
,
2017
Conformational Studies
https://doi.org/10.1038/nchem.2785
Binding kinetics in drug discovery
Molecular Informatics
,
2016
Protein-Ligand Binding
https://doi.org/10.1002/minf.201501018
Multibody cofactor and substrate molecular recognition in the myo-inositol monophosphatase enzyme
Scientific reports
,
2016
Protein-Ligand Binding
https://doi.org/10.1038/srep30275
HTMD: high-throughput molecular dynamics for molecular discovery
Journal of chemical theory and computation
,
2016
ACEMD/HTMD/AceCloud
https://doi.org/10.1021/acs.jctc.6b00049
The pathway of ligand entry from the membrane bilayer to a lipid G protein-coupled receptor
Scientific reports
,
2016
Membrane Proteins
https://doi.org/10.1038/srep22639
Insights from fragment hit binding assays by molecular simulations
Journal of chemical information and modeling
,
2015
Fragment Based Drug Discovery
https://doi.org/10.1021/acs.jcim.5b00453
AceCloud: molecular dynamics simulations in the cloud
Journal of Chemical Information and Modeling
,
2015
ACEMD/HTMD/AceCloud
https://doi.org/10.1021/acs.jcim.5b00086
Detection of new biased agonists for the serotonin 5-HT2A receptor: modeling and experimental validation
Molecular pharmacology
,
2015
Fragment Based Drug Discovery
https://doi.org/10.1124/mol.114.097022
HTMD: A complete software workspace for simulation-guided drug design
ABSTRACTS OF PAPERS OF THE AMERICAN CHEMICAL SOCIETY
,
2015
ACEMD/HTMD/AceCloud
https://doi.org/10.1021/acs.jctc.6b00049
Kinetic modulation of a disordered protein domain by phosphorylation
Nature communications
,
2014
Conformational Studies
https://doi.org/10.1038/ncomms6272
Kinetic characterization of fragment binding in AmpC β-lactamase by high-throughput molecular simulations
Journal of Chemical Information and Modeling
,
2014
Conformational Studies
https://doi.org/10.1021/ci4006063
Membrane lipids are key modulators of the endocannabinoid-hydrolase FAAH
Biochemical Journal
,
2014
Applications
https://doi.org/10.1042/BJ20130960
On-the-fly learning and sampling of ligand binding by high-throughput molecular simulations
Journal of chemical theory and computation
,
2014
ACEMD/HTMD/AceCloud
https://doi.org/10.1021/ct400919u
Reranking docking poses using molecular simulations and approximate free energy methods
Journal of chemical information and modeling
,
2014
Molecular Simulations
https://doi.org/10.1021/ci500309a
Identification of slow molecular order parameters for Markov model construction
The Journal of chemical physics
,
2013
ACEMD/HTMD/AceCloud
https://doi.org/10.1063/1.4811489
Kinetic characterization of the critical step in HIV-1 protease maturation
Proceedings of the National Academy of Sciences
,
2012
Conformational Studies
https://doi.org/10.1073/pnas.1211457109
High-throughput molecular dynamics: the powerful new tool for drug discovery
Drug discovery today
,
2012
Molecular Simulations
https://doi.org/10.1016/j.drudis.2012.03.017
Thumbs down for HIV: domain level rearrangements do occur in the NNRTI-bound HIV-1 reverse transcriptase
Journal of the American Chemical Society
,
2012
Conformational Studies
https://doi.org/10.1021/ja301565k
Visualizing the induced binding of SH2-phosphopeptide
Journal of chemical theory and computation
,
2012
Protein-Ligand Binding
https://doi.org/10.1021/ct300003f
Optimized potential of mean force calculations for standard binding free energies
Journal of Chemical Theory and Computation
,
2011
Applications
https://doi.org/10.1021/ct2000638
A high-throughput steered molecular dynamics study on the free energy profile of ion permeation through gramicidin A
Journal of Chemical Theory and Computation
,
2011
Applications
https://doi.org/10.1021/ct100707s
Complete reconstruction of an enzyme-inhibitor binding process by molecular dynamics simulations
Proceedings of the National Academy of Sciences
,
2011
Protein-Ligand Binding
https://doi.org/10.1073/pnas.1103547108
Explicit solvent dynamics and energetics of HIV‐1 protease flap opening and closing
Proteins: Structure, Function, and Bioinformatics
,
2010
Conformational Studies
https://doi.org/10.1002/prot.22806
Induced effects of sodium ions on dopaminergic G-protein coupled receptors
PLoS Computational Biology
,
2010
Membrane Proteins
https://doi.org/10.1371/journal.pcbi.1000884
High-throughput all-atom molecular dynamics simulations using distributed computing
Journal of chemical information and modeling
,
2010
Applications
https://doi.org/10.1021/ci900455r
ACEMD: Accelerating biomolecular dynamics in the microsecond time scale
Journal of Chemical Theory and Computation
,
2009
ACEMD/HTMD/AceCloud
https://doi.org/10.1021/ct9000685
An implementation of the smooth particle mesh Ewald method on GPU hardware
Journal of Chemical Theory and Computation
,
2009
Conformational Studies
https://doi.org/10.1021/ct900275y
The impact of accelerator processors for high-throughput molecular modeling and simulation
Drug discovery today
,
2008
Molecular Simulations
https://doi.org/10.1016/j.drudis.2008.08.001
International And EU Funded Projects
Cloud-HTMD
A cloud application platform for rational drug discovery using high throughput molecular dynamics.
European SME innovation Associate(H2020-INNOSUP-02-2016, Grant Agreement 739649)
CCFBLD-CHEMO
Computer-Centric Fragment Based Ligand Discovery for the Development of candidate molecules targeting the chemkine system.
Nuclis d’Innovació Tecnològica 2014. Acció, Generalitat de Catalunya. Nuclis Transnacionals Programa Bilateral Catalunya-Israel, Project nr. RDIS14-1-0002. 2014-2016
HTMD
Feasibility assessment of a cloud application platform for rational drug design using high-throughput.
H2020 SME Instrument 2014, Grant Agreement nr. 674659. 2015
CompBioMed
A Centre of Excellence in Computational Biomedicine.
H2020-EINFRA-2015-1, Grant Agreement nr. 675451. 2016-2019
CompBioMed2
A Centre of Excellence in Computational Biomedicine.
H2020-INFRAEDI-02-2018, Grant Agreement nr. 823712. 2019-2023